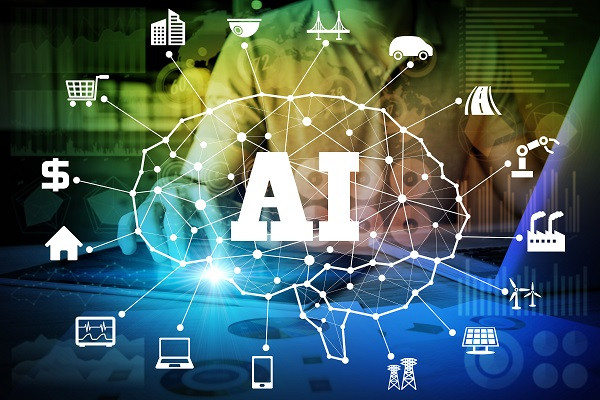
Artificial intelligence (AI) has rapidly advanced in recent years, with its applications expanding across various industries. However, behind the scenes of AI lies a crucial yet often overlooked factor: hardware.
This article delves into the world of AI hardware, focusing on popular processors and accelerators used in machine learning and neural networks. Additionally, it explores the memory requirements for efficient AI systems and analyzes the role of established tech giants like IBM in manufacturing AI hardware.
Finally, it discusses advancements and future trends in this ever-evolving field.
Key Takeaways
- AI processors and accelerators, such as NVIDIA Tesla V100, Google TPU, and Intel Movidius Myriad X, are crucial for efficient execution of machine learning algorithms and neural networks.
- Adequate memory capacity, including specialized hardware like GPUs and FPGAs, is crucial for efficient processing of neural network computations.
- Established tech giants like IBM play a crucial role in advancing AI hardware capabilities through heavy investment in research and development.
- Quantum computing holds potential for advancing AI capabilities, and edge computing complements AI hardware advancements by reducing latency and enhancing real-time decision-making.
AI Processors: Powering Machine Learning and Neural Networks
AI processors play a crucial role in enabling efficient execution of machine learning algorithms and neural networks. They serve as the backbone of machine learning algorithms, providing the computational power required to carry out complex calculations and data processing. These processors are specifically designed to handle the intensive workload associated with artificial intelligence tasks.
The impact of AI processors on neural network training is significant. Neural networks require massive amounts of computation to train and optimize their weights and biases. AI processors excel at performing parallel computations, which is essential for processing large datasets and optimizing neural networks efficiently. They enable faster training times by accelerating the computation process through specialized hardware architectures.
Leading tech giants like IBM have been actively involved in manufacturing AI hardware, including dedicated AI processors and accelerators. Their expertise in hardware design, coupled with their deep knowledge of artificial intelligence algorithms, allows them to develop cutting-edge solutions that meet the demanding requirements of modern AI applications. These advancements in AI hardware are instrumental in driving innovation and pushing the boundaries of what is possible in artificial intelligence research and development.
Boosting performance and efficiency, AI accelerators play a key role in the integration of hardware and artificial intelligence. These specialized processors are designed to handle the intensive computational tasks required by AI algorithms.
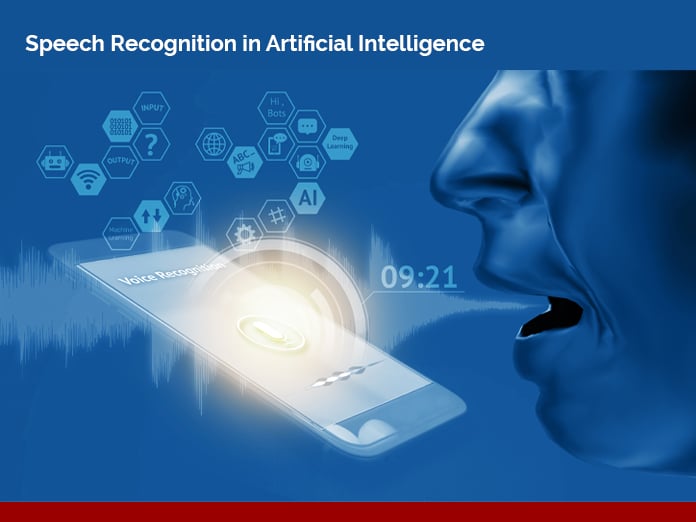
One popular type of AI accelerator is the Field-Programmable Gate Array (FPGA), which offers flexibility and reconfigurability for different applications. FPGAs can be programmed specifically for AI workloads, enabling faster processing speeds and lower power consumption compared to traditional CPUs or GPUs.
Another important component is the AI inference engine, which handles real-time decision-making based on trained models. These engines optimize performance by executing complex neural network computations efficiently.
Tech giants like IBM have been actively involved in developing AI hardware solutions, leveraging their expertise in both software and hardware domains. Their contributions have led to advancements in FPGA implementation and efficient AI inference engines, further driving progress in artificial intelligence technologies.
Memory Requirements for AI Systems
Memory requirements for AI systems are a crucial aspect that needs to be carefully considered in order to ensure efficient and effective processing of complex neural network computations. The amount of memory, particularly Random Access Memory (RAM), plays a significant role in determining the performance of AI systems.
As AI algorithms continuously process large amounts of data, it is essential to have sufficient memory capacity to store and access this data rapidly. Insufficient RAM can lead to bottlenecks, slowing down the processing speed and hindering real-time decision-making capabilities.
To address these challenges, specialized hardware such as Graphics Processing Units (GPUs) and Field-Programmable Gate Arrays (FPGAs) with dedicated memory architectures have emerged. These technologies enable parallel processing and high-bandwidth memory access, thereby enhancing AI system performance.
Additionally, advancements in non-volatile memory technologies like flash storage are being explored as they offer higher storage densities and lower power consumption compared to traditional RAM solutions.
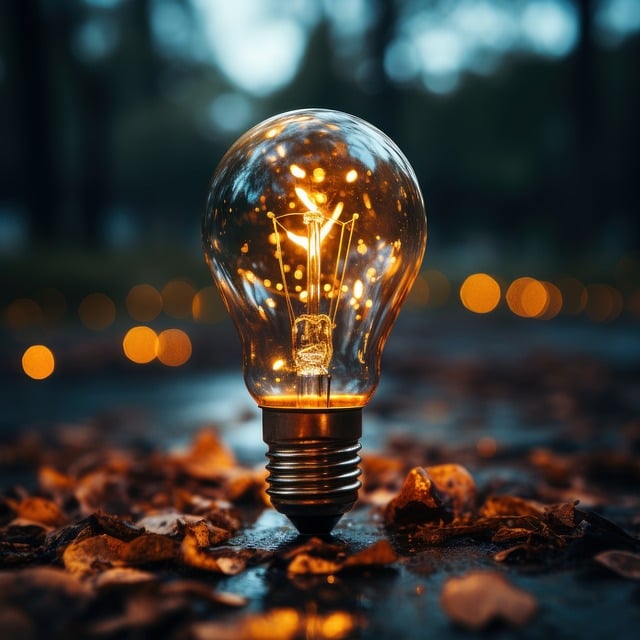
Sub-list 1: Inadequate memory capacity can hinder real-time decision-making capabilities
Sub-list 2: Specialized hardware like GPUs and FPGAs improve performance by enabling parallel processing
Sub-list 3: Advancements in non-volatile memory technologies show promise for enhanced storage density and reduced power consumption
Role of Tech Giants in AI Hardware Manufacturing
The involvement of major technology companies in the manufacturing of AI hardware is significant in shaping the landscape of this industry. Established tech giants like IBM play a crucial role in advancing AI hardware capabilities, including processors and accelerators designed specifically for artificial intelligence tasks. These companies invest heavily in research and development to create cutting-edge hardware solutions that can meet the increasing demand for AI processing power. One area where AI hardware has a substantial impact is the development of autonomous vehicles. The complex algorithms used in self-driving cars require powerful processors and memory systems to process vast amounts of data in real-time. Additionally, startups have emerged as disruptors in the AI hardware market, introducing innovative technologies and challenging established players with their unique offerings. Table 1 provides an overview of popular AI processors and accelerators.
Table 1: Popular AI Processors and Accelerators
Brand |
Processor/ Accelerator |
NVIDIA |
Tesla V100 |
Google |
Tensor Processing Unit (TPU) |
Intel |
Movidius Myriad X |
In conclusion, major tech giants like IBM are driving advancements in AI hardware manufacturing, enabling breakthroughs in various industries such as autonomous vehicles. Startups also play a vital role by introducing disruptive technologies that push boundaries and challenge established players. This active competition contributes to the continuous evolution of AI hardware, meeting the ever-growing demands for processing power required by modern artificial intelligence applications.
Advancements and Future Trends in AI Hardware
Advancements in AI hardware are transforming various industries and driving the evolution of processing power required by modern applications.
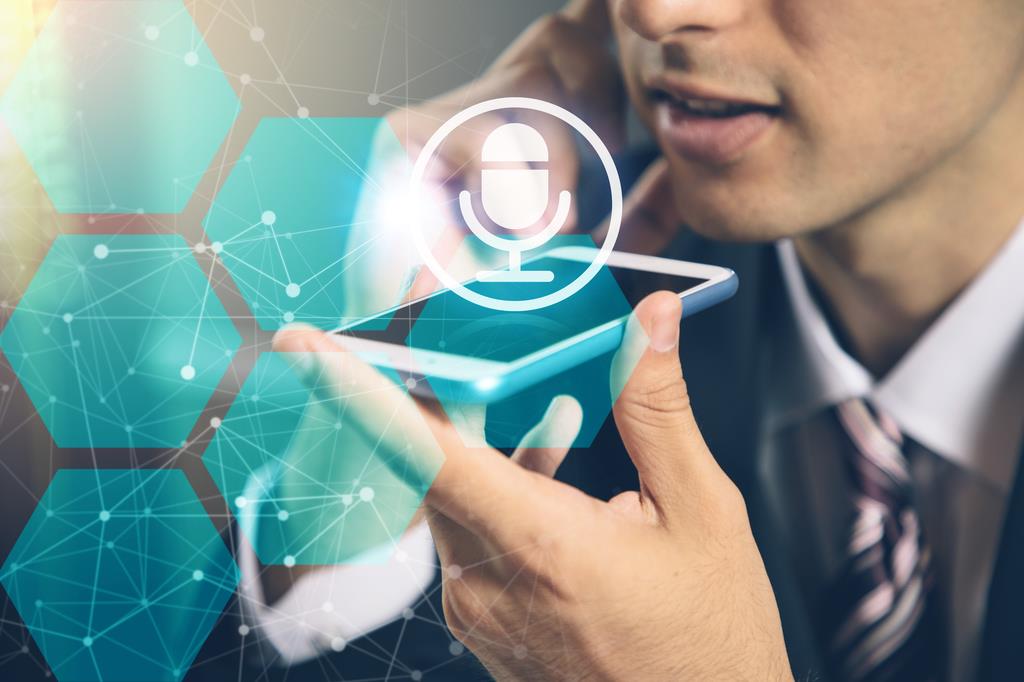
Quantum computing, with its ability to perform complex calculations at an unprecedented speed, holds immense potential for advancing AI capabilities. Its unique properties, such as superposition and entanglement, allow for parallel processing and solving computationally intensive problems efficiently.
Edge computing is another significant development that complements AI hardware advancements. It involves bringing computation closer to the data source, reducing latency and enhancing real-time decision-making capabilities. By processing data locally on edge devices instead of relying solely on cloud-based servers, edge computing enables faster response times and improved privacy.
The future of AI hardware lies in harnessing the power of quantum computing and integrating it with edge computing solutions. This combination can revolutionize various domains like healthcare, finance, and transportation by enabling more accurate predictions, efficient resource allocation, and enhanced security measures.
As researchers continue to explore these frontiers, we can expect further breakthroughs in AI hardware that will shape the future of artificial intelligence.
Frequently Asked Questions
What are the different types of AI processors available in the market?
The market offers various types of AI processors, each with its own performance and power consumption characteristics. These processors can be compared based on their ability to handle complex AI tasks while minimizing energy usage.
AI accelerators enhance the performance and efficiency of AI systems by offloading computationally intensive tasks from the main processor. This reduces energy consumption and enables faster processing, resulting in improved AI model training and inference capabilities.
What are the memory requirements for AI systems, and how do they vary based on the complexity of the AI model?
Memory requirements for AI systems vary based on the complexity of the AI model. More complex models require larger memory capacities to store and process data efficiently. The impact of model complexity on memory needs highlights the importance of adequate memory resources in AI hardware design.
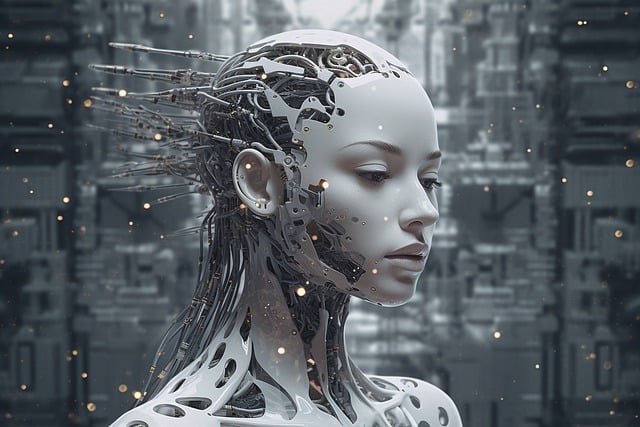
How are tech giants like IBM involved in AI hardware manufacturing?
Tech giants like IBM play a significant role in AI hardware manufacturing. They contribute by developing and producing advanced processors, accelerators, and memory systems that meet the complex requirements of AI models. IBM's expertise in this domain has enabled them to establish themselves as leaders in AI hardware production.
What are some of the latest advancements and future trends in the field of AI hardware?
Advancements in AI Hardware range from neuromorphic chips to quantum computing, revolutionizing the field. These advancements have a significant impact on edge computing, enabling faster processing and improved efficiency in AI applications.