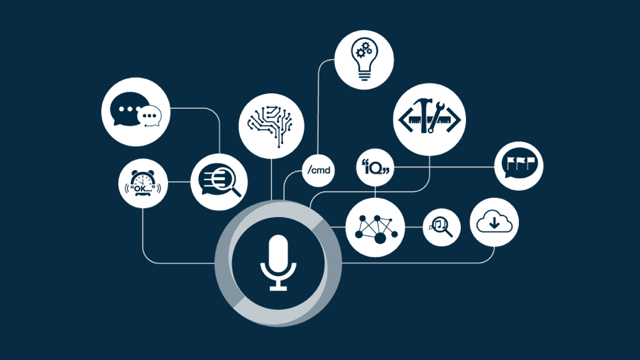
Machine learning and artificial intelligence (AI) have emerged as transformative technologies with wide-ranging applications in various industries.
This article explores the concept of machine learning, its different types, and its numerous uses across domains such as healthcare and natural language processing.
Additionally, it discusses the future scope of machine learning, highlighting advancements and opportunities that lie ahead.
Practical examples are provided to illustrate the practicality and advantages of this technology in business and research settings.
Key Takeaways
- Machine learning is a branch of artificial intelligence that involves creating algorithms and models that allow computers to learn and make predictions or decisions without being explicitly programmed.
- There are various types of machine learning algorithms, including supervised learning, unsupervised learning, image recognition, natural language processing, and fraud detection.
- Machine learning has a wide range of applications, such as healthcare (medical imaging analysis, predictive analytics, drug discovery), natural language processing (customer service, automated translation), finance (risk minimization, efficiency improvement), transportation (autonomous vehicles, traffic management), agriculture (crop yield prediction, pest detection), and education (adaptive learning platforms, personalized tutoring).
- The future of machine learning holds great potential for advancements in fields like robotics, finance, efficiency improvement, risk minimization, and offers limitless possibilities.
Types of Machine Learning Algorithms
There are several types of machine learning algorithms that can be classified based on their learning approach and the type of data they process.
One such type is supervised learning, where the algorithm learns from labeled training data to make predictions or decisions. This approach requires a clear distinction between input variables and output variables.
On the other hand, unsupervised learning algorithms do not rely on labeled data but instead identify patterns and structures within the dataset. They aim to discover hidden relationships or groupings in the data without any prior knowledge.
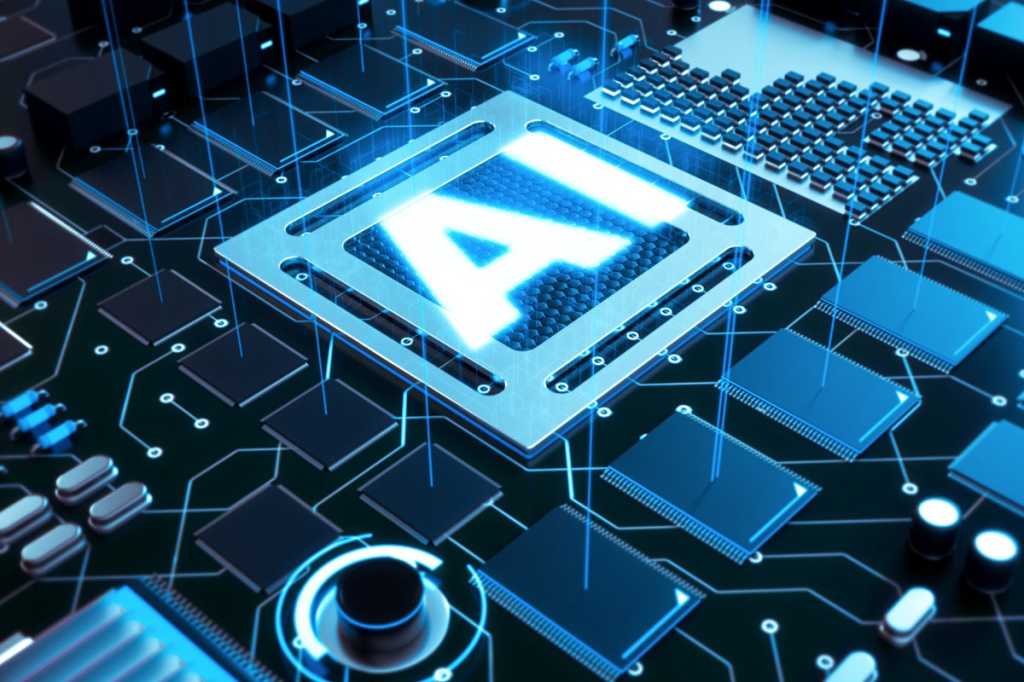
These algorithms are widely used in various applications such as image recognition, natural language processing, recommendation systems, fraud detection, and many more.
Understanding these different types of machine learning algorithms is crucial for effectively applying them in real-world scenarios and harnessing their potential benefits.
Applications of Machine Learning in Healthcare
The application of machine learning in healthcare involves the utilization of algorithms and data analysis techniques to improve medical diagnostics, treatment plans, and patient outcomes. Machine learning has wide-ranging applications in this field, including:
Medical imaging analysis: Machine learning algorithms can analyze medical images, such as X-rays or MRIs, to detect abnormalities or assist in diagnosis.
Predictive analytics: By analyzing large datasets, machine learning can predict patient outcomes and identify individuals at risk of developing certain diseases.
Drug discovery: Machine learning models can help identify potential drug candidates by analyzing molecular structures and predicting their effectiveness.
Electronic health records (EHR): Machine learning algorithms can extract valuable insights from EHRs, such as identifying patterns for disease progression or suggesting personalized treatment plans.
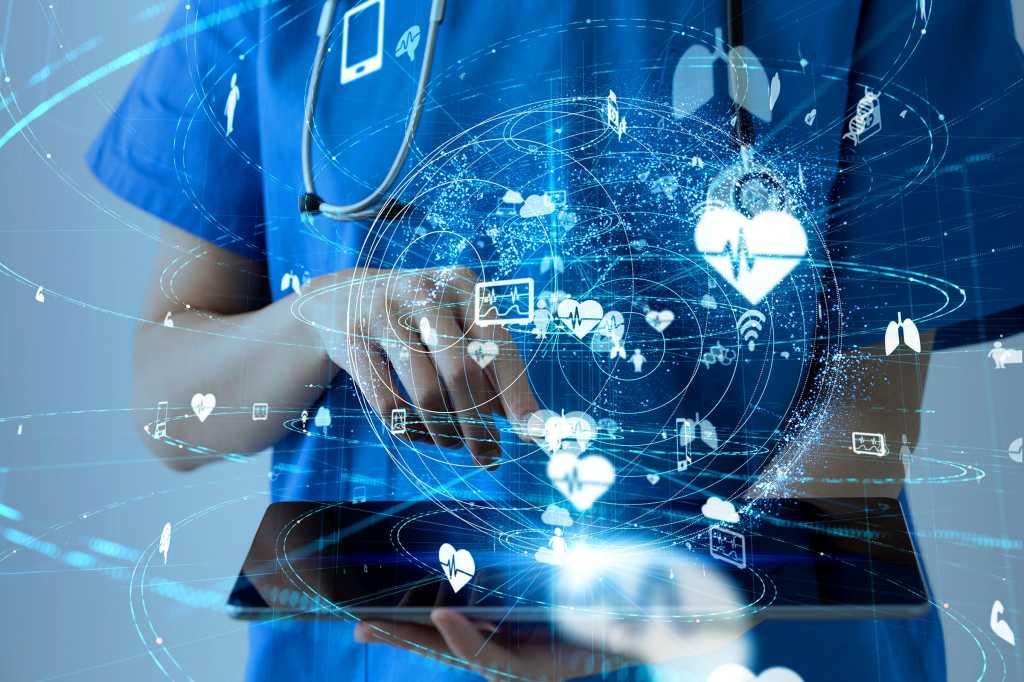
In addition to healthcare, machine learning finds applications in finance, transportation, and logistics sectors as well. The scope for further advancements is promising as technology continues to evolve rapidly in these areas.
Natural Language Processing: Enhancing Communication with Machines
Natural Language Processing (NLP) is a field of study that focuses on enhancing communication between humans and machines by developing algorithms and techniques to understand, interpret, and generate human language.
NLP has numerous applications in improving customer service and automated translation. For customer service, NLP enables machines to analyze customer queries and provide accurate responses, reducing the need for human intervention. This improves efficiency and allows businesses to handle large volumes of inquiries effectively.
Additionally, NLP plays a crucial role in automated translation systems by enabling machines to understand the context, grammar, and semantics of different languages. This facilitates accurate translation between languages, making it easier for people from diverse linguistic backgrounds to communicate effectively.
The advancements in NLP have revolutionized communication with machines by bridging the gap between human language understanding and machine processing capabilities.
The Future of Machine Learning: Advancements and Opportunities
Advancements and opportunities in machine learning are driving the future of AI, with potential applications ranging from healthcare to finance and beyond. Machine learning algorithms have shown promise in revolutionizing robotics by enabling autonomous decision-making and enhancing human-robot interactions. In the field of finance, machine learning techniques are being utilized to analyze vast amounts of financial data, identify patterns, and make accurate predictions for investment strategies. These advancements offer opportunities to improve efficiency, reduce costs, and minimize risks in various industries.
In order to evoke an emotional response in the audience, a table showcasing the transformative impact of machine learning can be incorporated:
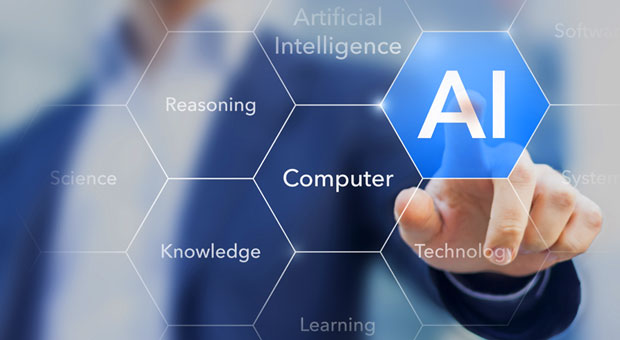
Application |
Impact |
Healthcare |
Improved diagnosis accuracy; personalized treatment options |
Transportation |
Safer autonomous vehicles; optimized traffic management |
Agriculture |
Enhanced crop yield prediction; precision farming |
Education |
Adaptive learning platforms; personalized tutoring |
Customer Service |
Chatbots for improved customer interactions; sentiment analysis |
The future scope of machine learning is extensive as it continues to evolve and find new applications across diverse domains. The possibilities are limitless as technology advances and data availability increases, providing further opportunities for innovation.
Advantages of Machine Learning in Business and Research
One key benefit of incorporating machine learning in business and research is the ability to analyze large volumes of data quickly and accurately. In the field of finance, machine learning algorithms can process vast amounts of financial data to identify patterns, predict market trends, and make informed investment decisions. This enables businesses to optimize their portfolios, minimize risks, and maximize profits.
Moreover, machine learning offers numerous benefits in manufacturing by optimizing production processes, reducing downtime, improving quality control, and predicting maintenance needs. By analyzing historical data and real-time sensor information, machine learning algorithms can identify anomalies or potential failures before they occur, enabling proactive measures to be taken. These advantages not only enhance operational efficiency but also contribute to cost savings in terms of reduced waste and increased productivity.
Overall, the incorporation of machine learning in business and research brings significant advantages by leveraging its ability to analyze large volumes of data quickly and accurately for informed decision-making.
Frequently Asked Questions
How does machine learning differ from traditional programming?
Machine learning differs from traditional programming in that it allows systems to learn and improve from data without explicit programming instructions. This approach offers benefits such as the ability to handle complex tasks, adaptability, and scalability for a wide range of applications.
What are some limitations or challenges of implementing machine learning in healthcare?
Limitations and challenges of implementing machine learning in healthcare include data privacy concerns, lack of interpretability, limited availability of high-quality datasets, and the need for regulatory compliance. Implementation challenges involve integrating ML systems with existing healthcare infrastructure and ensuring clinical acceptance.
Can machine learning be used to detect and prevent fraudulent activities in the financial sector?
Machine learning applications in finance have proven effective for fraud detection and prevention. By analyzing large datasets, machine learning algorithms can identify patterns and anomalies associated with fraudulent activities, enabling timely intervention to protect financial institutions and customers.
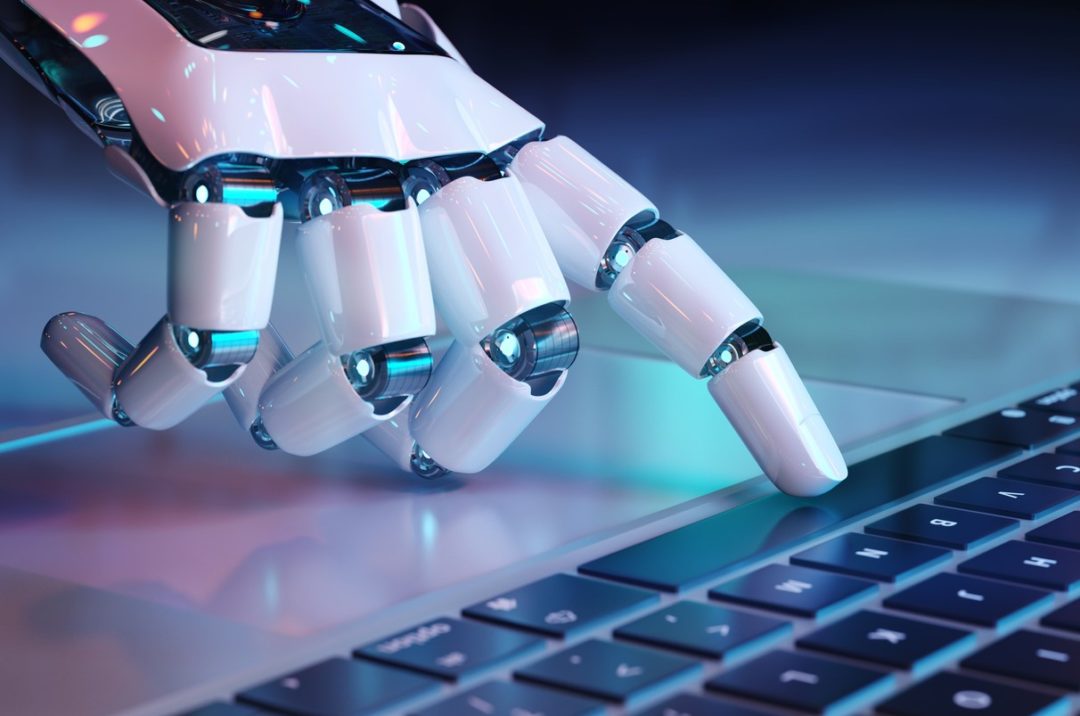
How does natural language processing improve the accuracy of voice assistants like Siri or Alexa?
Natural Language Processing (NLP) improves voice assistants' accuracy by enhancing natural language understanding and improving voice recognition accuracy. NLP techniques, such as speech recognition and language modeling, enable better interpretation of user commands and provide more accurate responses.
What are some potential ethical concerns or risks associated with the future advancements of machine learning?
Ethical implications and privacy concerns are major considerations in the future advancements of machine learning. The potential misuse of personal data, algorithmic bias, and autonomous decision-making raise questions about accountability, transparency, and fairness in AI systems.